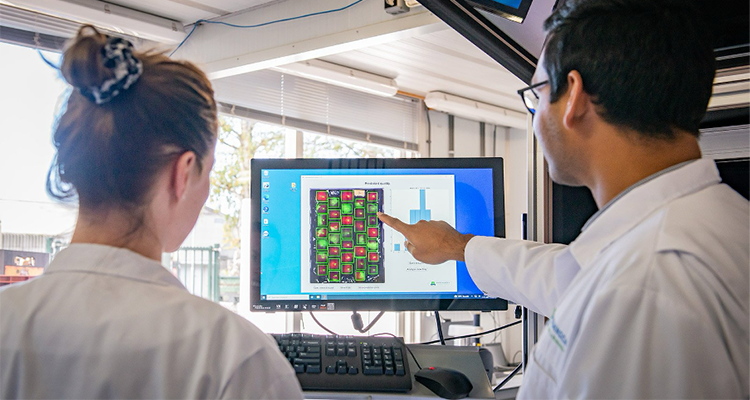
Thesis subject
Hyperspectral Imaging for Non-destructive Food Quality Assessment (BSc/MSc)
In this thesis, we embark on a journey through the world of hyperspectral imaging and cutting-edge machine learning. Our focus is on transforming the way food quality is assessed, making use of advanced non-destructive measurement tools. Previous research has shown that hyperspectral imaging makes it possible to accurately predict a multitude of food quality attributes in a variety of food products and agriculture materials.
Short description
This thesis aims to contribute to ongoing research on hyperspectral imaging as an innovative approach to assess food quality. Many essential properties of fresh food, such as Brix value, dry matter content, internal damage, and firmness, remain invisible to the naked eye. Spectrometers and hyperspectral cameras enable non-destructive measurement of these properties. This thesis focuses on establishing models to predict these intrinsic quality parameters using spectral data. As this technology becomes increasingly cost-effective, faster, and suitable for industrial applications, it holds a great promise for automated quality control and sorting of large volumes of food.
Objectives and tasks
The following research objectives can be explored:
- Hyperspectral image processing
- Working with all in one spectral imaging system
- Regression and classification analysis for model development with hyperspectral imaging
- Deep learning for hyperspectral imaging such as segmentation, object detection, and predictive modelling
Literature
- Mishra, P., Sytsma, M., Chauhan, A., Polder, G., & Pekkeriet, E. (2022). All-in-one: a spectral imaging laboratory system for standardised automated image acquisition and real-time spectral model deployment.Analytica Chimica Acta,1190, 339235. https://doi.org/10.1016/j.aca.2021.339235
- Amigo, J. M., Babamoradi, H., & Elcoroaristizabal, S. (2015). Hyperspectral image analysis. A tutorial. Analytica chimica acta, 896, 34-51. https://doi.org/10.1016/j.aca.2015.09.030
Requirements
- Courses: Programming in Python (INF-22306), Statistics (MAT), Big Data (INF-33806) or Machine Learning (FTE-35306) or Sensing and Perception (FTE27306)
- Required skills/knowledge: Experience in data analytics and willingness to learn new data-driven tools, general interest in the (agri)food sector
Key words: Data Science, Machine Learning, Food Quality Assessment, Hyperspectral imaging, Sensors, Non-destructive.
Contact person(s)
Sander Breevaart (sander.breevaart@wur.nl)
Puneet Mishra (puneet.mishra@wur.nl)