Project
Modeling Social Dynamics with Complex Systems; Emergent effects of Governance on Agriculture, by Scaling the local behavior of individual cellular automata, using Utility Maximization strategies, Markov chains and Adoption behavior
Although the past few decades have allowed for success in attempts to model land-use, successful modeling of social dynamics remains elusive. For example, interactions between expansion of agricultural area, intensification and governance are still poorly understood across spatial scales.
Empirical work allowing for increased understanding of these interactions is very scarce. However, in my recent global study (The role of governance in agricultural expansion and intensification: a global study of arable agriculture, 2011), statistical relationships between expansion of arable agricultural area, intensification and governance were found at the national level. In my present work I make an attempt to reproduce these relationships with a land-use model that I have constructed. The model is a complex system constructed in Netlogo, based on utility maximization, Markov chains and adoption behavior (fig 1).
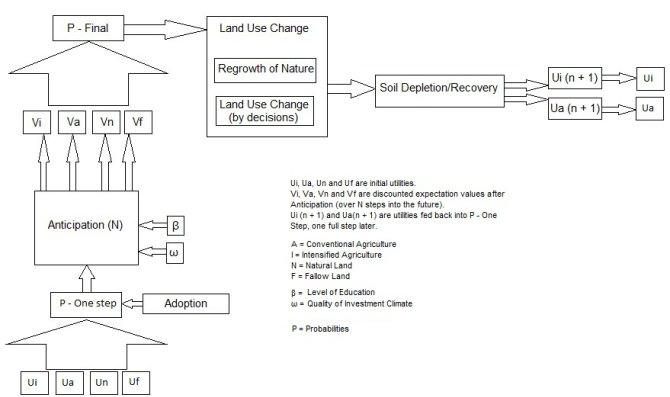
The model consists of ca. 10,000 interacting cellular automata that maximize utility (gained from transitions), exhibiting preferences for intensive agriculture, conventional agriculture, natural land or fallow land. The model can be related and calibrated to real-world data, through generated land-use patterns (fig 2, 5) and available quantitative data on used governance indicators (World Bank). Furthermore, the model can import spatially (positively) auto-correlated land-use-preference maps, containing a realistic distribution of utilities associated with the different land-uses. I included those indicators of governance from the empirical work I wish to examine, that could be modeled within the framework of decision dynamics theory; namely “government effectiveness” and “regulatory quality” (World Bank). Furthermore, I include these at firm level, as local conditions provided for by government. “Government effectiveness” refers to the provision, by government agencies, of public goods and services (and quality thereof), in particular of governmental agricultural research and education programs. “Regulatory quality” expresses how well private sector development is promoted by government, i.e. the quality of investment climate. “Government effectiveness” is assumed to be modeled by the degree of rationality of decisions, bounded by the level of education. “Regulatory quality” is assumed to be modeled by allowing decision horizons of firms to vary from a minimum short term horizon of one step(yr); low foresight, high risk, to a maximum long term horizon of 10 steps(yrs); high foresight, low risk. These varying decision horizons are assumed to reflect a varying level of uncertainty within an investment climate.
The overall degree of uncertainty of the system can be measured statistically, by calculating the information-entropy over the decision-space (land-use transitions), and entire system of ca. 10,000 automata. This is the most natural and objective way to characterize large-scale probabilistic system behavior, and it reveals conceptual (in)consistencies with respect to system state variables. An important general result predicted by the model, is that when information-entropy is minimized for a land-use system, gains in utility are maximized. Due to less stochastic (and thus more ordered/intelligent) outcomes of individual strategies for lower entropy. Analogous to thermodynamics: the amount of disorder of a closed system (zero external energy input) is maximized in the macroscopic equilibrium state. E.g. an ice-cube slowly melting and evaporating. Initially, it’s entropy is minimized (in frozen state), gradually increasing until it’s molecules are (probabilistically) uniformly distributed over the volume the ice-cube was in. We would have to add energy to the ice-cube, to keep it in a state of minimized entropy. Furthermore, the model predicts that entropy is nearly-minimized at nearly-maximized quality of governance, and vice versa, consistently. Thus, we have that gains in utility are maximized for nearly-maximized quality of governance, and minimized for nearly-minimized quality of governance. The relations between entropy, landscape and system state gain variables over the governance-parameter space, are illustrated clearly by fig 2-7, for the system states of maximum and minimum entropy, while the system had a preference for agriculture. Without assuming non-linearity (adoption behavior), coherent landscapes and consistent entropy relations with respect to governance-parameters and utility do not emerge. They are truly emergent properties, in the sense of complex systems. Furthermore, a viable system-wide transition from conventional to intensive agriculture emerges over a specific range of ‘good’ governance.
Note that when the quality of investment climate exceeds ca. 0.4, the entropy remains nearly-minimal for both the maximum and minimum entropy scenario, in the direction of the investment climate. A system-wide transition from conventional to intensive agriculture ‘wants’ to occur from 0 to 0.4; beyond we have stability. However, for the maximum entropy scenario (fig 2-4), the entropy remains nearly-maximal in the direction of level of education. I.e. the level of education is too low to sustain a system-wide transition from conventional to intensive agriculture. This explains the decreasing gains (fig 3). For the minimum entropy scenario, the entropy remains nearly-minimal in both directions from 0.4 onwards, and the system-wide transition persists.
Fig 2-4. Maximum Entropy: landscape (yellow is conventional agriculture, black is fallow land, red is intensive agriculture and green is nature); utility gains for near-minimum constant level of education and increasing quality of investment climate (I=intensive agriculture, A=conventional agriculture); and entropy itself, for near-minimum constant level of education and increasing quality of investment climate. Points of maximum entropy are indicated by the black box.
Fig 5-7. Minimum Entropy: landscape (yellow is conventional agriculture, black is fallow land, red is intensive agriculture and green is nature); utility gains for maximum constant level of education and increasing quality of investment climate (I=intensive agriculture, A=conventional agriculture); and entropy itself, for maximum constant level of education and increasing quality of investment climate. Points of minimum entropy are indicated by the black box.