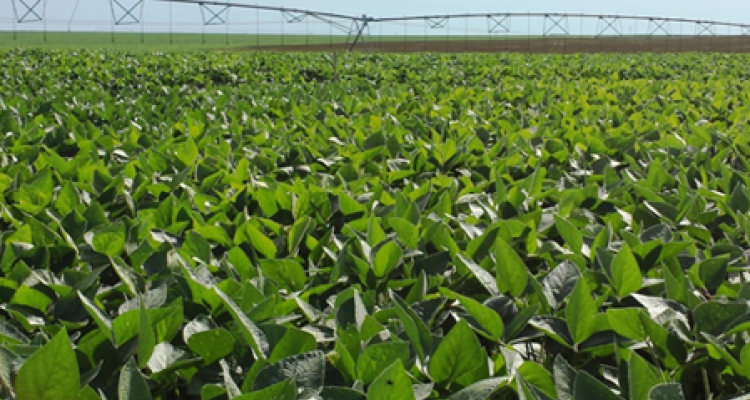
Colloquium
Predicting Soybean Within-Field Yield by Coupling Evapotranspiration from Landsat-Based Metric Into a Crop Growth Model
By Vorayuth Pakachaipong
Abstract
Reliable and timely within-field yield variability prediction is necessary to optimize crop yield and minimize the environmental impact of crop production. Evapotranspiration (ET) could be estimated from satellite imagery but is challenging to assimilate into a crop growth model for within-field yield variability prediction because of its non-state variable nature. In this study, the potential of assimilating Landsat-based METRIC (Mapping EvapoTranspiration at high Resolution with Internalized Calibration) into a crop growth model for within-field soybean yield variability prediction was evaluated over two fields. First, ET was estimated from two Landsat 8 imagery over a growing season with the pyMETRIC tool. Second, an existing soybean growth model was modified. Third, a particle filter was developed to assimilate the estimated ET into the modified soybean growth model. Last, the results were evaluated against yield observation collected from a yield monitor system. The data assimilation showed to be able to assimilate spatial variability observed in the ET estimation into the prediction but poorly predicted within-field spatial variability when compared with the yield observation. The relative root means square error was 11% for the first field and 5.5% for the second field. The within-field yield prediction accuracy was comparable to the assimilation of the Leaf Area Index (LAI) into a soybean growth model done in the previous study over the same study area and period. Though, the accuracy of within-field yield spatial variability prediction was likely limited by the low number of ET observations and the insensitive of a crop growth model to ET. Future work should aim to address these two points or to jointly assimilate LAI and ET into a crop growth model to investigate the potential accuracy improvement from multivariate data assimilation.