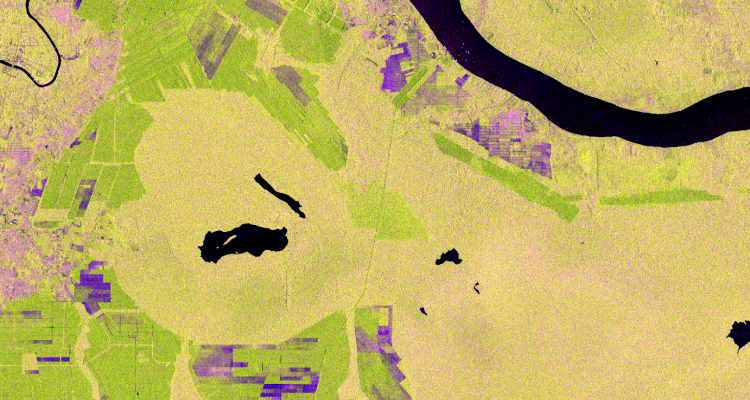
Radar Remote Sensing
Our research utilizes radar remote sensing for unravelling human activities in and dynamics of forest ecosystems at regional to global scales, and has a strong focus on near real-time change monitoring. We develop machine learning methods using radar and multi-sensor data to monitor change dynamics, their drivers and associated greenhouse gas emissions more accurately and timely.
Satellite radar remote sensing uses long-wavelength energy that penetrates through clouds and is sensitive to changes in vegetation physical structure. These characteristics are major advantages for monitoring forest dynamics, in particular in tropical regions where cloud cover is persistent. New and near-future radar satellites provide spatially detailed information almost daily and cloud computing solutions now enable large-scale applications.
An alarming rate of human-induced forest loss combined with more frequent extreme climate events strongly increases the pressure on the Earth’s forest ecosystems. At the same time, countries and major commodity sectors commit to zero-deforestation pledges. We work on openly accessible and timely information on the dynamics and drivers of changes in forests urgently needed to empower sustainable land management, law enforcement actions against illegal activities, and climate change law implementations.
Our core research lines are:
- Fundamental radar methods
- Near real-time change monitoring
- Characterising forest changes and dynamics
Current PhD, Postdoc and research projects
- Dr. Maciej Soja (Researcher): Biomass and selective logging monitoring using high resolution radar
- Dr. Robert Masolele (Postdoc): Large scale forest dynamics and driver monitoring
- Dr. Laura Cué la Rosa (Postdoc): Near-future deforestation prediction using deep learning
- Dr. Johannes Balling (Postdoc): Characterizing tropical forest change dynamics using multi-sensor satellite data
- Bart Slagter (PhD Student): Mapping and characterisation of small-scale forest disturbance using satellite data and artificial intelligence
- Anne-Juul Welsink (PhD student): Monitoring selective logging using advanced earth observation datasets
- Sietse van der Woude (PhD student): European forest monitoring linking satellite and ground-based data streams
- Karimon Nesha (PhD student): Progressing the use of earth observation
and national in-situ data for monitoring of forest emissions - Arjen de Jonge (PhD student): Detection of drought effects on trees using near- and remote-sensing
Former colleagues
- Dr. Adugna Mullissa (Postdoc 2018 - 2022) - continued at CTrees, United States
- Dr. Christelle Braun (Postdoc 2018 - 2020) - continued at UNEP, Senegal
- Dr. Wanda de Keersmaecker (Postdoc 2019 – 2021) - continued at VITO, Belgium
- Dr. Ovidiu Csillik (Postdoc 2019-2021) - continued at JPL, USA
- Dr. Yaqing Gou (Postdoc 2020 – 2022) – continued at Rabobank, The Netherlands
- Dr. Milutin Milenkovic (Postdoc 2018 – 2022) – continued at IIASA, Austria
Key Publications
Fundamental radar methods
-
Seasonal Forest Disturbance Detection Using Sentinel-1 SAR & Sentinel-2 Optical Timeseries Data and Transformers
In: IGARSS 2023 - 2023 IEEE International Geoscience and Remote Sensing Symposium - IEEE - ISBN: 9798350331745 -
Despeckling Polarimetric SAR Data Using a Multistream Complex-Valued Fully Convolutional Network
IEEE Geoscience and Remote Sensing Letters (2022), Volume: 19 - ISSN 1545-598X - p. 1-5. -
deSpeckNet: Generalizing Deep Learning-Based SAR Image Despeckling
IEEE Transactions on Geoscience and Remote Sensing (2022), Volume: 60 - ISSN 0196-2892 - p. 1-15. -
Large Area Aboveground Biomass and Carbon Stock Mapping in Woodlands in Mozambique with L-Band Radar: Improving Accuracy by Accounting for Soil Moisture Effects Using the Water Cloud Model
Remote Sensing (2022), Volume: 14, Issue: 2 - ISSN 2072-4292 -
Sentinel-1 SAR Backscatter Analysis Ready Data Preparation in Google Earth Engine
Remote Sensing (2021), Volume: 13, Issue: 10 - ISSN 2072-4292 -
Angular-Based Radiometric Slope Correction for Sentinel-1 on Google Earth Engine
Remote Sensing (2020), Volume: 12, Issue: 11 - ISSN 2072-4292 -
Dual Polarimetric SAR Covariance Matrix Estimation Using Deep Learning
-
Polsarnet: A deep fully convolutional network for polarimetric sar image classification
IEEE Journal of Selected Topics in Applied Earth Observations and Remote Sensing (2019), Volume: 12, Issue: 12 - ISSN 1939-1404 - p. 5300-5309. -
Multi-model radiometric slope correction of SAR images of complex terrain using a two-stage semi-empirical approach
Remote Sensing of Environment (2015), Volume: 156 - ISSN 0034-4257 - p. 1-10.
Near real-time change monitoring
-
Integrating satellite-based forest disturbance alerts improves detection timeliness and confidence
Environmental Research Letters (2024), Volume: 19, Issue: 5 - ISSN 1748-9326 -
Deep learning and automatic reference label harvesting for Sentinel-1 SAR-based rapid tropical dry forest disturbance mapping
Remote Sensing of Environment (2023), Volume: 298 - ISSN 0034-4257 -
How textural features can improve SAR-based tropical forest disturbance mapping
International Journal of applied Earth Observation and Geoinformation (2023), Volume: 124 - ISSN 1569-8432 -
Inter-comparison of optical and SAR-based forest disturbance warning systems in the Amazon shows the potential of combined SAR-optical monitoring
International Journal of Remote Sensing (2023), Volume: 44, Issue: 1 - ISSN 0143-1161 - p. 59-77. -
Rapid remote monitoring reveals spatial and temporal hotspots of carbon loss in Africa’s rainforests
Communications Earth & Environment (2022), Volume: 3, Issue: 1 - ISSN 2662-4435 -
Forest disturbance alerts for the Congo Basin using Sentinel-1
Environmental Research Letters (2021), Volume: 16, Issue: 2 - ISSN 1748-9318 -
Near-daily discharge estimation in high latitudes from Sentinel-1 and 2: A case study for the Icelandic Þjórsá river
Remote Sensing of Environment (2020), Volume: 241 - ISSN 0034-4257 -
Characterizing Tropical Forest Cover Loss Using Dense Sentinel-1 Data and Active Fire Alerts
Remote Sensing (2018), Volume: 10, Issue: 5 - ISSN 2072-4292 -
Improving near-real time deforestation monitoring in tropical dry forests by combining dense Sentinel-1 time series with Landsat and ALOS-2 PALSAR-2
Remote Sensing of Environment (2018), Volume: 204 - ISSN 0034-4257 - p. 147-161. -
Combining satellite data for better tropical forest monitoring
Nature Climate Change (2016), Volume: 6, Issue: 2 - ISSN 1758-678X - p. 120-122.
Characterising changes and dynamics
-
Mapping the diversity of land uses following deforestation across Africa
Scientific Reports (2024), Volume: 14 - ISSN 2045-2322 -
Monitoring direct drivers of small-scale tropical forest disturbance in near real-time with Sentinel-1 and -2 data
Remote Sensing of Environment (2023), Volume: 295 - ISSN 0034-4257 -
Towards the use of satellite-based tropical forest disturbance alerts to assess selective logging intensities
Environmental Research Letters (2023), Volume: 18, Issue: 5 - ISSN 1748-9318 -
Alert-Driven Community-Based Forest Monitoring: A Case of the Peruvian Amazon
Remote Sensing (2022), Volume: 14, Issue: 17 - ISSN 2072-4292 -
Intra-annual relationship between precipitation and forest disturbance in the African rainforest
Environmental Research Letters (2022), Volume: 17, Issue: 4 - ISSN 1748-9318 -
Exploring Archetypes of Tropical Fire-Related Forest Disturbances Based on Dense Optical and Radar Satellite Data and Active Fire Alerts
Forests (2021), Volume: 12, Issue: 4 - ISSN 1999-4907 -
Linking Terrestrial LiDAR Scanner and Conventional Forest Structure Measurements with Multi-Modal Satellite Data
Forests (2019), Volume: 10, Issue: 3 - ISSN 1999-4907 -
Characterizing Tropical Forest Cover Loss Using Dense Sentinel-1 Data and Active Fire Alerts
Remote Sensing (2018), Volume: 10, Issue: 5 - ISSN 2072-4292
Other related publications
-
Critical Assessment of Cocoa Classification with Limited Reference Data: A Study in Côte d’Ivoire and Ghana Using Sentinel-2 and Random Forest Model
Remote Sensing (2024), Volume: 16, Issue: 3 - ISSN 2072-4292 -
Assessing Amazon rainforest regrowth with GEDI and ICESat-2 data
Science of Remote Sensing (2022), Volume: 5 - ISSN 2666-0172 -
Glasgow forest declaration needs new modes of data ownership : Monitoring progress in the Glasgow ‘Declaration on Forests’ remains impossible without open sharing of data. Three actions are required if this declaration is to succeed.
Nature Climate Change (2022), Volume: 12, Issue: 5 - ISSN 1758-678X - p. 415-417. -
Evaluating recovery metrics derived from optical time series over tropical forest ecosystems
Remote Sensing of Environment (2022), Volume: 274 - ISSN 0034-4257 -
Improving Forest Baseline Maps in Tropical Wetlands Using GEDI-Based Forest Height Information and Sentinel-1
Forests (2021), Volume: 12, Issue: 10 - ISSN 1999-4907 -
Intra-Annual Identification of Local Deforestation Hotspots in the Philippines Using Earth Observation Products
Forests (2021), Volume: 12, Issue: 8 - ISSN 1999-4907 -
Mapping wetland characteristics using temporally dense Sentinel-1 and Sentinel-2 data: A case study in the St. Lucia wetlands, South Africa
International Journal of applied Earth Observation and Geoinformation (2020), Volume: 86 - ISSN 1569-8432 -
The Role and Need for Space-Based Forest Biomass-Related Measurements in Environmental Management and Policy
Surveys in Geophysics (2019), Volume: 40, Issue: 4 - ISSN 0169-3298 - p. 757-778. -
A review of the application of optical and radar remote sensing data fusion to land use mapping and monitoring
Remote Sensing (2016), Volume: 8, Issue: 1 - ISSN 2072-4292
Available open-source software
- GEE code to integrate various alert datasets (e.g. RADD, GLAD-L, and GLAD-S2) using approach published in Reiche et al., (2024). https://code.earthengine.google.com/?accept_repo=users/nrtwur/alert_integration
- 'Bayts' SEPAL implementation: Open-access implementation of the BayTS Sentinel-1 alerts (RADD-like alerts) at the SEPAL platform. A step-by-step guide is available (2023).
- Sentinel-1 Analysis Ready Data GEE repository: Code for Sentinel-1 SAR Backscatter Analysis Ready Data Preparation in Google Earth Engine (Mullissa et al, 2021): https://github.com/adugnag/gee_s1_ard.
- ‘Bayts’ R-package: https://github.com/jreiche/bayts.
Set of tools to apply the probabilistic machine learning approach of Reiche et al. (2015, 2018) to combine multiple Radar and/or optical satellite time series and to detect deforestation/forest cover loss in near real-time. - Code for Angular-based radiometric slope correction of Sentinel-1 on Google Earth Engine (Vollrath et al 2020): https://github.com/ESA-PhiLab/radiometric-slope-correction.
Code for deSpeckNet (Mullissa et al, 2020): Generalizing Deep Learning Based SAR Image Despeckling: https://github.com/adugnag/deSpeckNet (original Matlab code) | https://github.com/adugnag/deSpeckNet-TF-GEE (python implementation to seamlessly integrate Sentinel-1 SAR image preparation in GEE with deep learning in Tensorflow for SAR image despeckling).
- Code for CV-deSpeckNet (Mullissa et al, 2021) - Despeckling Polarimetric SAR Data Using a Multistream Complex-Valued Fully Convolutional Network: https://github.com/adugnag/CV-deSpeckNet.