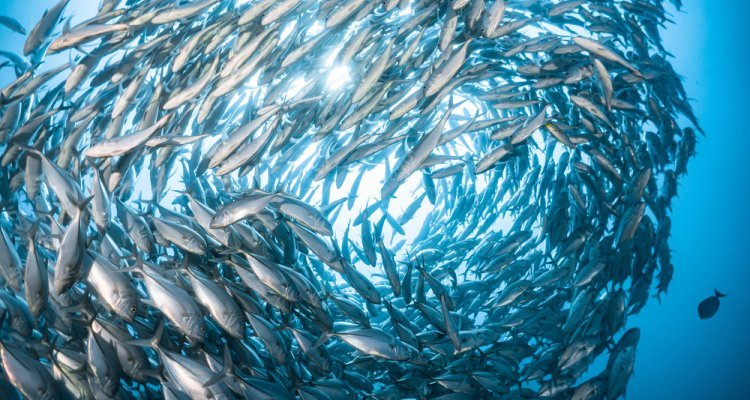
Project
Improved quality assurance of image analyses for assessments
A key input of fisheries stock assessment models is the age structure of a fish population, which has important implications for recruitment processes, population fluctuations, and ultimately catch options advice for fisheries management. Currently, the main method of determining the age of a fish relies on manually counting the annual growth rings in otoliths, but this process is labour intensive and depends on specialist expertise.
The process to read the age from otoliths is as follows; (1) the otoliths are collected from the fish, then (2) are sliced and photographed, and finally, (3) an expert age reader examines the photographed otolith to count the annual growth rings (usually winter rings) and thereby the age. At Wageningen Marine Research (WMR) the age reading is done by age-reading specialists, which manually annotate otolith images using the software tool SmartDots. However, sometimes otolith age rings are difficult to identify, and counts are then somewhat subjective. Different expert readers might produce different results, requiring internationally coordinated quality assessments as well as calibration exercises.
Recent advances in machine learning have provided deep learning methods that are remarkably successful in automating the process of labour-intensive manual analysis of counting various objects. Especially in digital image analysis tasks, deep learning provides new opportunities for optimizing analytical workflows. It is possible that the otoliths that are harder to read for humans are also harder to read with an computer algorithm. However, it is expected that any potential bias in automated readings will have a consistent bias, instead of one that varies per observer and through time. One of the main aims of this project is to determine the differences in the values, errors, consistencies and biases between automated and human age readings.