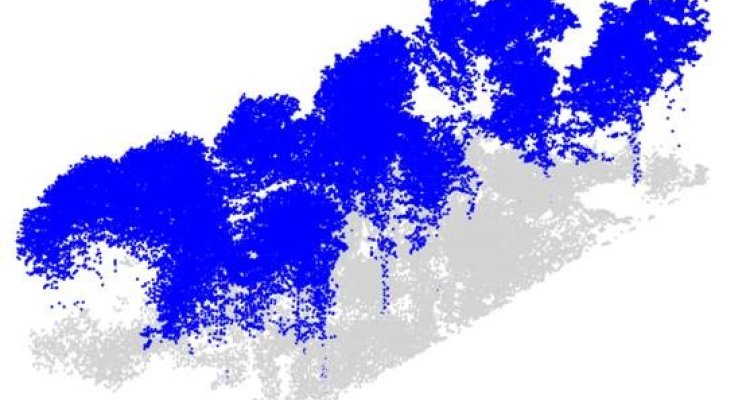
Colloquium
AHN for monitoring succession processes in forest structure
By Tijs de Pagter
Abstract
The increase in homogeneity of stand structures in temperate forests over the past decades has led to a decrease in biodiversity in temperate forests. This underlines the need for research into measures for forest management which could improve biodiversity. In forest monitoring and research, Airborne Laser Scanning (ALS) is increasingly being used to obtain information on forest structure. In the Netherlands, the Actueel Hoogtebestand Nederland (AHN) is a country-wide ALS dataset, originally created by a collaboration of provincial governments and waterboards for hydrology and water management purposes. However, the use of the AHN for forest monitoring remains largely unexplored. This thesis investigated the suitability of the AHN for the monitoring of changes in forest structure, in particular in areas where a decrease in canopy cover through forest management practices has occurred. To do so, this thesis aimed to develop a forest structure mapping procedure which could be used with AHN point clouds. First, a literature review was conducted, which investigated the different methods for mapping forest structure with ALS data that exist in literature. These methods were then assessed on suitability with the AHN through a theoretical and practical test, and from this methods were selected, and a procedure was developed. The procedure was tested on a case study in two patches of forest in the south of the Dutch province of Limburg, and which the effect of reduced canopy cover on forest structure was investigated. The literature review found that current methods for forest structure mapping could be divided into four categories, being: ALS-derived metrics, vertical stratification, segmentation algorithms, and deep learning. The results of the developed procedure were visually assessed and were found to generally perform well. However, there were some errors caused by over-segmentation, and errors caused by dense overstory vegetation. It was concluded that to improve the procedure, further calibration of the methods was necessary. Furthermore, the procedure should be tested further in different forest types to assess its performance outside the case study areas.