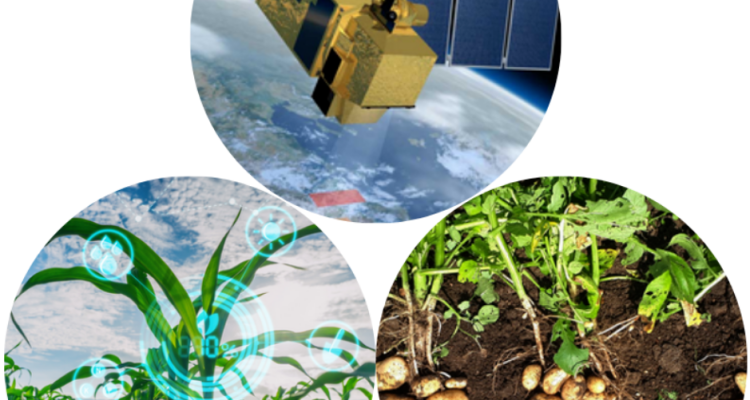
Colloquium
Improving WOFOST biomass and yield simulations: Assimilating leaf area index and canopy nitrogen content with the Ensemble Kalman filter
By Lucas Rivero
Abstract
The growing world population, food security and climate change, will force changes in food systems to ensure a sustainable and livable Earth. Accurate predictions of biomass and yield at the field level are crucial for effective agricultural monitoring, crop management, and the formulation of food policies aimed at closing yield gaps. Process based crop growth models offer a tool to predict biomass and yield by considering environmental and management variables, thereby providing decision makers with updated model information. Remote sensing derived biophysical variables can complement process based models by assimilating remote sensing data to guide them towards a more realistic state. Variables such as leaf area index (LAI) and canopy nitrogen content (CN), retrievable through remote sensing techniques, are essential for simulating biomass and yield. However, the selection of the most suitable crop growth model for accurately predicting potato biomass and yield using in situ canopy state data remains uncertain due to insufficient data and experimentation. Additionally, the performance of assimilating canopy variables through ensemble Kalman filter techniques into crop growth models has not yet been tested to evaluate their capabilities in spatializing biomass and yield at the field level. With the advancement of the WOFOST crop growth model, capable of simulating biomass and yield, and the availability of high spatiotemporal remote sensing data from Sentinel 2, assimilating remotely derived biophysical variables into crop growth models can significantly enhance crop biomass and yield simulations. In this study, leaf area index and canopy nitrogen content data were assimilated into the WOFOST model using the ensemble Kalman filter algorithm to improve biomass and yield simulation for potato agrosystems in the Netherlands at the field level. Evaluation was conducted to determine which WOFOST version simulated best biomass and yield when assimilating leaf area index and/or canopy nitrogen content measurements. Assimilating only leaf area index showed the best results, with WOFOST 7.2 considering simulating biomass and yield. It yielded a median R2 = 0.39 and 0.88, ME = -136.40 and 1220.01 kg/ha, RMSE = 566.64 and 1837.23 kg/ha, RRMSE = 0.33 and 0.23, and PBIAS = 6.91 and -14.29 % for biomass and yield respectively, across all 68 treatments studied. This represented a more accurate and less biased model compared to a non-data assimilation scheme for biomass. However, none of the data assimilation setups improved yield simulations compared to the non-data assimilating schema, showing more research is needed to improve remote sensing aided crop yield simulations. For computing remote sensing derived leaf area index, the Clre spectral index was selected due to its better alignment with the development of leaf area index throughout the growing season. Assimilating remote sensing derived leaf area index into WOFOST 7.2, to simulate final yield at the national scale, resulted in ME = 429.24 kg/ha, RMSE = 2716.5 kg/ha, RRMSE = 0.21, and PBIAS = 3.39 % across all 90 fields studied, representing a reduction of 1343.23 kg/ha for the mean error and a 10.59 % reduction in bias compared to the non-assimilation scheme. Hence, assimilating remote sensing derived leaf area index into WOFOST 7.2 improved yield predictions, offering a methodology to improve potato crop yield simulations.