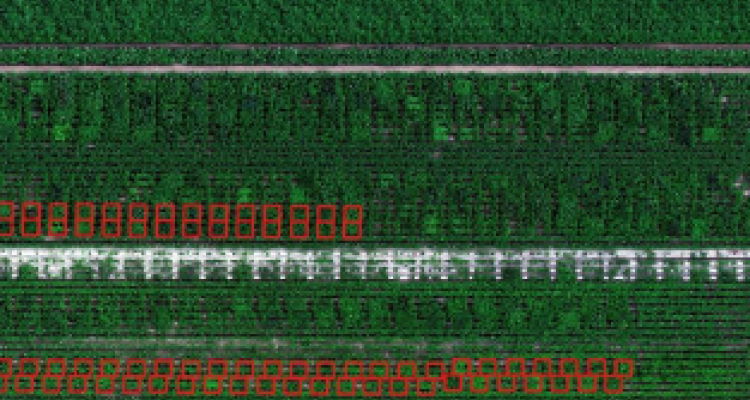
Colloquium
Analysis of UAV Time-series in Phenotyping applications in Potato with Double Logistic Curve Fitting
By Simon Bosselaar
Abstract
The global population continues to grow, while climate and environmental-related issues are increasing. This amplifies the urgency for developing new plant varieties for food production. In plant breeding, monitoring crop phenology is key in identifying genotypes with desired growth characteristics. Current plant phenotyping is done manually and is time-consuming, labour-intensive and subjective. Therefore, there is a need for high-throughput phenotyping systems that process large amounts of data in a short time. Satellite-based phenotyping offers a solution, but is limited by its low spatial resolution. Unmanned Aerial Vehicle (UAV) based phenotyping presents the opportunity to solve these problems. In this study, multispectral UAV time series are used to explore the potential of using the vegetation indices (VIs) NDVI, NDRE, WDVI and CIRED to model the growth curve of a potato crop and show differences in treatment or genotypes. Modelling of the growth curve was done by fitting a double logistic function on the vegetation indices (VI) and the model parameters Start of Season (SOS), growth rate at SOS (rSOS), End of Season (EOS), growth rate at EOS (rEOS), minimum (MIN) and maximum (MAX) were derived. After manually adding winter baseline values, all VIs, but CIRED, resulted in good quality curve fits. SOS showed the highest correlation with the field observations and yield, with NDVI derived SOS being the highest. Most of the other parameters showed weak correlations. NDRE showed to have the capacity to distinguish groups with a different nitrogen treatment after applying a principal component analysis. However, it is important to perform enough observations throughout the growing season and include winter values for a good quality curve fit. This could also increase the correlations of model parameters with field observations. Also, it would be useful to test this methodology on multiple growing seasons to generalise the findings. Overall, this study showed the potential of using UAV time series for high-throughput phenotyping in potatoes, but also emphasizes further research.