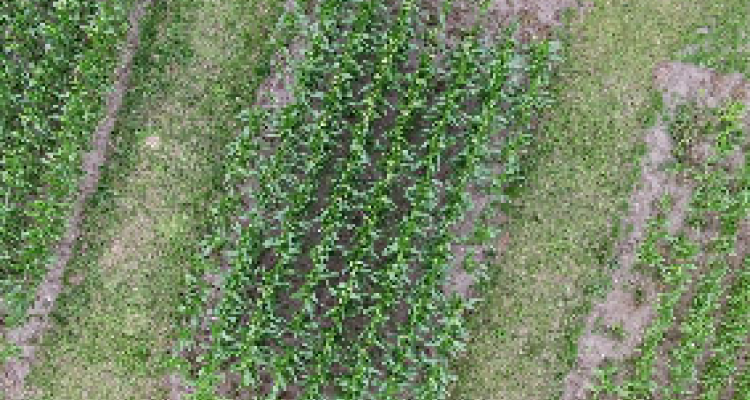
Colloquium
Estimating Plant Nitrogen Content in Maize using Multispectral UAV Data
By Ilse Radix
Abstract
Nitrogen is one of the most important nutrient for agricultural crops to obtain optimal growth and yield. To increase the available nitrogen in the soil, often artificial fertiliser is used. However, negative ecological implications can arise when too much nitrogen is applied. Alternative methods to increase the available soil nitrogen are investigated, one of which is the planting of cover crops in winter time. Experiments on the effects of alternative nitrogen fertilizers on the plant nitrogen concentration (PNC) requires accurate measurements. Current methods are destructive and expensive. A cheap and non-destructive method is the use of remote sensing. This research will investigate what is the best method to measure PNC in summer maize using multispectral UAV images. Since 2016, a field experiment has been conducted with vetch oat, radish and combinations growing on fixed plots in winter time. Each summer different summer crops have been planted. In 2022, the summer crop was maize. The performance of linear regression, lasso regression, random forest and gradient boosting for PNC prediction were compared. A total of 23 vegetation indices on top of the 5 spectral indices were tried as input to the model. It was investigated whether the addition of a crop height model could improve the models. The performance of PNC predictions was compared with the predictions of leaf nitrogen content (LNC). Lasso regression and random forest performed equally. PNC and LNC were predicted with a coefficient of determination of 0.71 and 0.8 respectively. Blue, red, MCARI and VARI were selected as most important variables by the model. Addition of a crop height model, slightly decreased the performance of the models, due to faulty DSM’s. The PNC model was not able to distinguish the differences between winter crop treatments. This research shows that PNC can be measured using multispectral UAV images and lasso regression or random forest. However, the model is not accurate enough to spot differences in PCN between different winter crop treatments. The results shows potential for monitoring of alternative fertilisation experiments using remote sensing.